The CHPC has a new page summarizing machine learning and artifical intelligence resources.
Center for High Performance Computing
Research Computing and Data Support for the University Community
In addition to deploying and operating high-performance computational resources and providing advanced user support and training, CHPC serves as an expert team to broadly support the increasingly diverse research computing and data needs on campus. These needs include support for big data, big data movement, data analytics, security, virtual machines, Windows science application servers, protected environments for data mining and analysis of protected health information, advanced networking, and more.
If you are new to the CHPC, the best place to learn about CHPC resources and policies is our Getting Started page.
Have a question? Please check our Frequently Asked Questions page and contact us if you require assistance or have further questions or concerns.
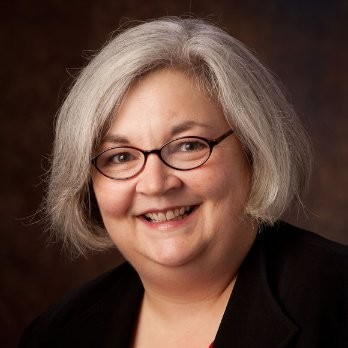
After nearly four decades of dedicated service at the University of Utah, Julia Harrison is retiring as the Operations Director of the Center for High Performance Computing.
Read more
Anita M. Orendt is a dedicated educator and researcher with a rich background in physical chemistry. Anita has made significant contributions to the academic community at the University of Utah.
Read moreUpcoming Events:
Allocation Requests for Winter 2025 are Due December 1st, 2024
Posted November 4th, 2024
Update to redwood idle session management following August 20, 2024 downtime
Posted September 3rd, 2024
Redwood Cluster Operating System Updated to Rocky Linux 8.10
Posted August 21st, 2024
Allocation Requests for Fall 2024 are Due September 1st, 2024
Posted August 7th, 2024
Allocation Requests for Summer 2024 are Due June 1st, 2024
Posted May 1st, 2024
CHPC Downtime: Tuesday March 5 starting at 7:30am
Posted February 8th, 2024
Two upcoming security related changes
Posted February 6th, 2024
Allocation Requests for Spring 2024 are Due March 1st, 2024
Posted February 1st, 2024
CHPC ANNOUNCEMENT: Change in top level home directory permission settings
Posted December 14th, 2023
CHPC Spring 2024 Presentation Schedule Now Available
CHPC PE DOWNTIME: Partial Protected Environment Downtime -- Oct 24-25, 2023
Posted October 18th, 2023
CHPC INFORMATION: MATLAB and Ansys updates
Posted September 22, 2023
CHPC SECURITY REMINDER
Posted September 8th, 2023
CHPC is reaching out to remind our users of their responsibility to understand what the software being used is doing, especially software that you download, install, or compile yourself. Read More...News History...
An Essential Need for HPC in Nuclear Engineering Applications
By Meng-Jen (Vince) Wang, and Glenn E. Sjoden
Department of Civil and Environmental Enginnering, University of Utah
The University of Utah’s Nuclear Engineering Program (UNEP) has greatly benefitted from the tremendous resources afforded for research by the University of Utah’s Center for High Performance Computing (CHPC). The modern reality is that nuclear applications cannot be practically fielded without an extraordinary amount of modeling and simulation carried out prior to actual testing, evaluation, or new engineering endeavor. For this reason, we must maintain access to “premier” computing resources, such as those available via CHPC, simply because models that require detailed radiation transport simulations are computationally intense. Overall, radiation transport simulations consist of two main approaches: deterministic solvers that require solutions of many billions of simultaneous equations, or Monte Carlo based solvers that require billions of particle histories to simulate, in addition to proper pre-processing of nuclear data to enable complex system computations. UNEP maintains HPC parallel optimized codes that can utilize both deterministic and Monte Carlo solution approaches; in fact, most often, we must apply both approaches to validate our findings to ensure all nuances of profiling “where the radiation goes” is fully explored. This is where having the resources enabled by CHPC is essential; these HPC frameworks are comprehensively pressed to execute extremely accurate models, enabling us to achieve what others may not be able to, allowing us to proceed with novel research objectives with the knowledge that we can successfully achieve our end goals.
For example, using both NOTCHPEAK and REDWOOD at CHPC, we have successfully executed simulations of highly accurate 3-D models of the University of Utah Training Reactor (UUTR), as shown in the above figure. Based on these results, we were able to complete the design of new neutron beam port and source configurations in our reactor, and complete a new beam stop and imaging chamber design. In addition, the simulations enabled us to design, optimize, and perform testing in our UUTR reactor to support a new methodology for Xe-135 gas standards for calibration studies used by the Comprehensive Test Ban Treaty Organization (CTBTO).
For more information, see our Spring 2022 newsletter here.
System Status
General Environment
General Nodes | ||
---|---|---|
system | cores | % util. |
kingspeak | 868/952 | 91.18% |
notchpeak | 2673/3212 | 83.22% |
lonepeak | 1906/1932 | 98.65% |
Owner/Restricted Nodes | ||
system | cores | % util. |
ash | Status Unavailable | |
notchpeak | 21193/22068 | 96.03% |
kingspeak | 2858/5244 | 54.5% |
lonepeak | 0/416 | 0% |
Protected Environment
General Nodes | ||
---|---|---|
system | cores | % util. |
redwood | 378/628 | 60.19% |
Owner/Restricted Nodes | ||
system | cores | % util. |
redwood | 1860/6444 | 28.86% |